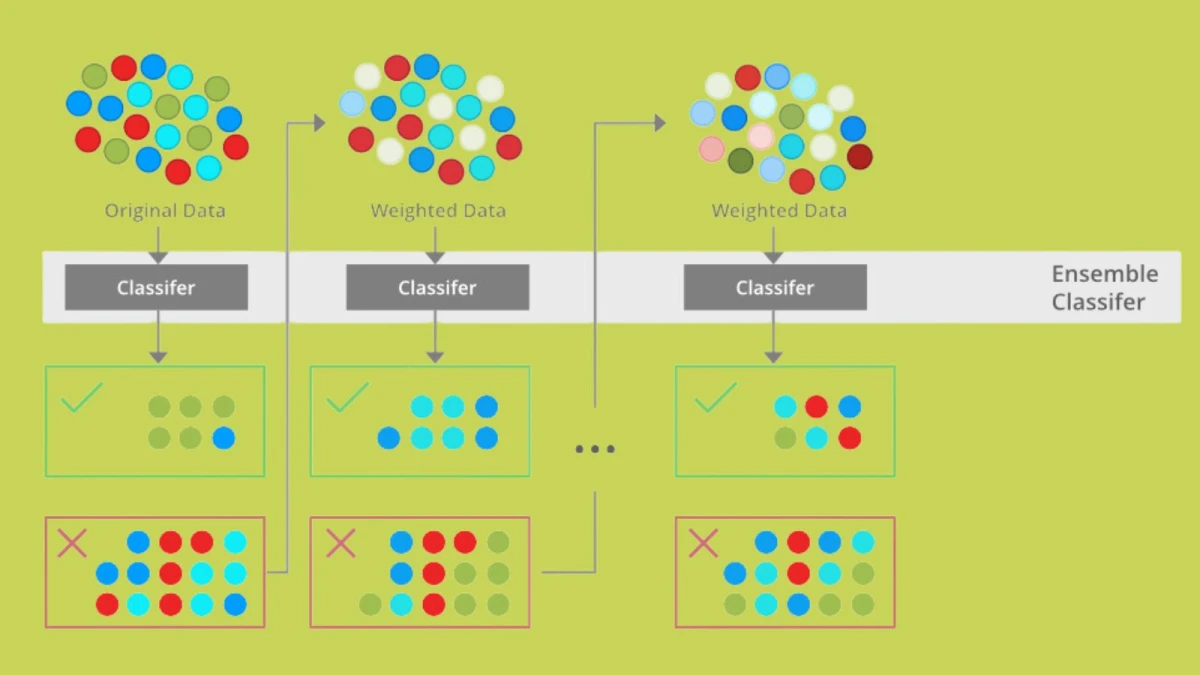
Crowd pleaser: Ensemble modelling for accuracy and explainability
At the heart of every predictive modelling exercise is the accuracy-explainability dichotomy, which can pull actuaries in different directions. Traditional models such as generalised linear models (GLMs) have long been favoured for their simplicity and transparency, but the quest for better performance has introduced more complex algorithms, such as gradient boosting machines (GBMs).
Practitioners often feel forced to opt for one or the other, depending on whether interpretability or predictiveness is the primary goal. However, the approach need not be so binary: a wide array of architectures combine the two.
Combining multiple model predictions into one is known as ensembling, with ‘bagging’ and ‘boosting’ being the standard techniques. Our goal is to use these combinations to improve predictive performance while retaining as much explainability as possible.
Bagging
Bagging, short for ‘bootstrap aggregating’, involves randomly sampling with replacement from the data, and training a model on each of these bootstrapped samples. For a regression task, the final prediction is the average of all the model predictions.
[....]